Achieving Returns in an Accelerating AI Environment
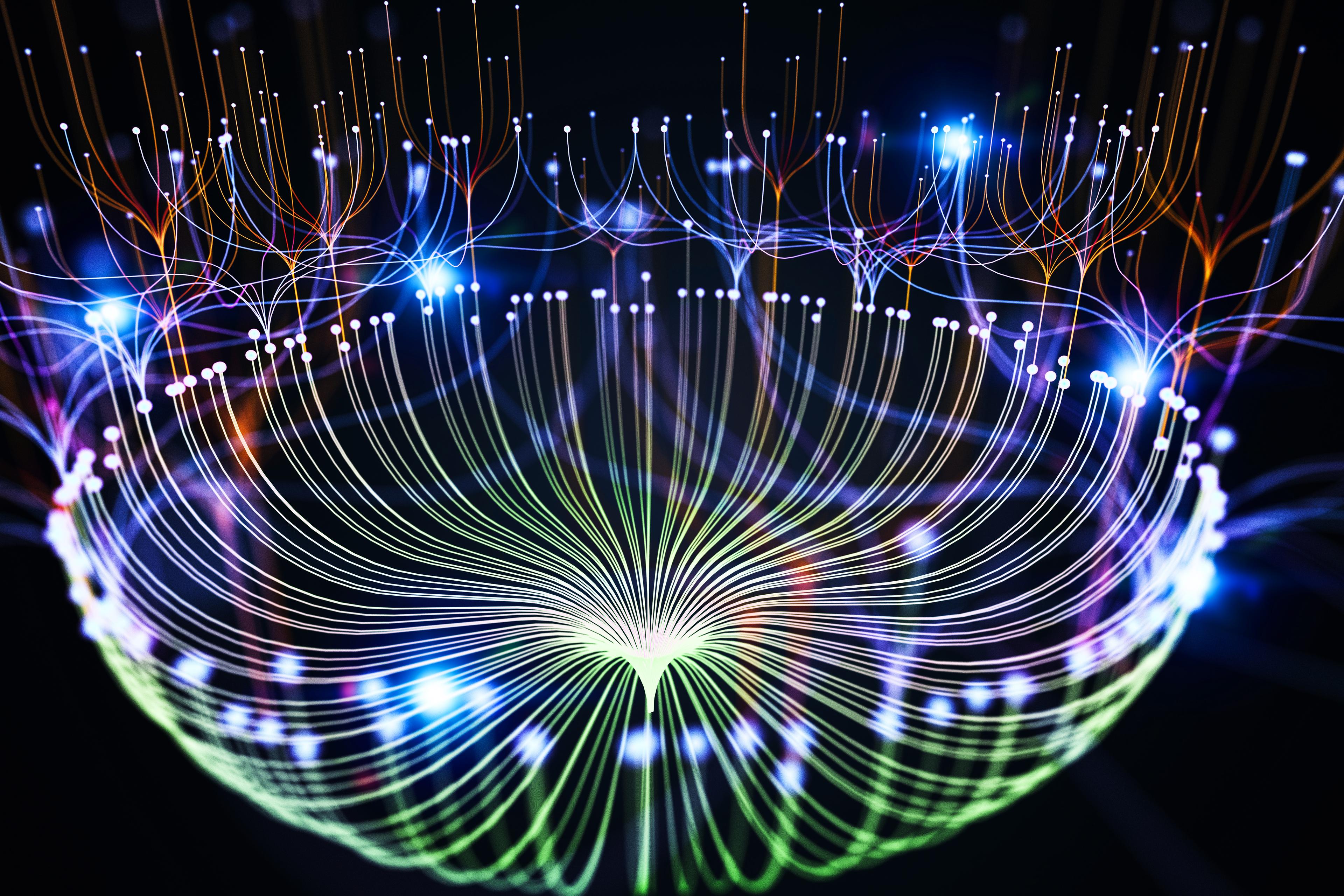
From improvements in Machine Learning and the development of new database systems to the development of sector-specific tools, significant advances have taken place in artificial intelligence (AI).
Faced with a backdrop of an uncertain economic outlook, however, some businesses might be slowing down investments in AI, which we think would be a mistake.
Those companies may risk being left behind in an ever-digitizing world where adopting AI and subsequent modernization of the organizational tech infrastructure has become, quite simply, table stakes for businesses to remain competitive. The use cases and returns regarding organizational AI-based tool adoption are vast and only increasing - time and cost savings, productivity and revenue increases, improvement in experience, retention and organizational agility, to name a few.
We use our experience as a leading Technology Investment Bank to spend our time advising companies on how to reach their strategic objectives.1 A large part of this involves strategies around raising and deploying capital in the segments of the technology sector with the greatest potential for growth.
Despite the current backdrop of challenging capital markets and even layoffs among some technology companies, we still see AI accelerating. In fact, based on what I’m currently hearing from clients and seeing in the industry, including startups and more established technology companies that are rapidly innovating, we expect the next decade to be a leading one for mass AI adoption.
Below are key themes and trends which have allowed the generation of significant ROI through AI.
1. Deep Learning Models Revolutionizing the Future of AI
Although the purpose of this piece is not to do a deep dive into the history of AI, it is important to note that we are indeed only at the beginning of truly feeling the impact of this technology in our lives. Though many people have only recently become familiar with this technology, the theory goes back several decades. Warren McCulloch and Walter Pitts talked about the concept of an artificial neural network as early as 1943. While the groundwork for the field was being laid in the 1950s and 1960s, it was not until the late 1990s that the power of Deep Learning technology was truly recognized. Yann Le Cun’s 1998 seminal paper on the use of artificial neural networks for image recognition was one such paper. Today, Le Cun and two other industry giants, Geoffrey Hinton and Yoshua Bengio, are deservedly considered the godfathers of Deep Learning and were bestowed the Turing Award in 2018. The field continues to evolve through the contributions of many other brilliant minds in academia and business actively working to make Machine Learning methods even more effective.
Prior to the advent of Deep Learning, the Machine Learning process was done by supervised training of the algorithm through a series of examples to learn from, which required data to be structured and labeled. Through Deep Learning, the algorithms can analyze incredible volumes of unstructured data and identify the features, links or interactions between certain concepts without supervision.
As models continue to evolve and computing power has become less of a limiting factor, AI has become more and more accessible to the masses. Percolating out of university research labs, to initially large deep-pocketed tech companies, and now in organizations of all sizes. Currently, AI, Machine Learning and Deep Learning models are being utilized to analyze vast volumes of data to solve and optimize many business problems, and we are only at the beginning!
Tip on optimizing ROI: Not every problem requires the most complex model. Understand your business needs and limitations in utilizing AI models to analyze data or solve business problems.
2. New Sophisticated Databases Enabling AI Business Applications
As AI models have evolved and become more sophisticated and capable of learning from large amounts of unstructured data, so have the database needs of organizations to better leverage this paradigm shift.
By utilizing a purpose-built database, organizations can significantly speed up the Machine Learning process and the training of these models. In addition, by using specific databases, organizations can better handle volume, velocity, and complexity of data governance and management challenges associated with Machine Learning to save time and optimize resources.
At the cutting edge of the database technology is the integration of AI with Database Management System (DBMS) technologies which promise to shape the future of computing. This integration is crucial not only for next-generation computing but also for the continued development of database technology. While both AI and DBMS systems represent well-established fields, research and development in AI/DB integration is still in its infancy. The motivations driving the integration of these two technologies include the need for speedy access to large amounts of shared data for efficient management and intelligent data processing. These autonomous databases use Machine Learning to automate database tuning, security, backups, updates and other routine management tasks traditionally performed by administrators. Unlike a conventional database, an autonomous database performs all these tasks, and more, without human intervention.
The ultimate value of an AI database is in optimizing computing and database resources. This lets you create better Machine and Deep Learning models, train them faster and more efficiently, and maintain a thorough line of how AI will be applied to your business.
Tip on optimizing ROI: Understand your overall organization’s Line of Business specific database requirements, then layer on the AI considerations to optimize for needs and resourcing.
3. Proliferation of Verticalized Solutions
This part of the AI revolution should have been well anticipated. As technologies mature and become increasingly accessible to the masses and diverse market needs, there is always a phase of proliferation in verticalized and point solutions. We saw the same phenomenon in Marketing Technology during the rise of Social Media and Big Data and in Cybersecurity with the increase in cyberattacks, for example. History repeats itself as we see the same phenomenon in AI and database technologies. More accessible Machine and Deep Learning models combined with increasingly sophisticated database technology have allowed for the proliferation of verticalized or point solutions addressing specific needs. Why? Verticalized or specialized point solutions have a better-defined and replicable ROI, allowing for penetration of a focused market. Furthermore, this targeted Sales and Marketing effort tends to improve the efficiency of customer acquisition and shorten sales cycles. Rinse and repeat.
AI-based solutions leveraging modern database technology are here to stay and are already having a significant impact on our lives. Natural Language Processing allows us to converse with our fridges about which groceries to order, while chatbots have become human-like customer service representatives. Robotic Process Automation has taken over repetitive, mundane tasks to alleviate employees’ time for more important responsibilities. The consumer experience has improved significantly through hyper-personalization of the consumer journey. Cybersecurity tools have emerged to help information security teams reduce breach risk and improve their security posture efficiently and effectively.
Tip on optimizing ROI: Smaller organizations that do not have the capacity for dedicated AI and DBMS resources can utilize out-of-the-box point solutions with much lower investment requirements. Identify whether AI-based tools to meet your needs already exist before trying to build your own internal solutions.
Burgeoning Investment: Of course, moving AI forward requires capital, and while tech investments have more broadly slowed down in 2022 in anticipation of a slowing economy, there’s still plenty of appetite for AI. According to estimates from Research and Markets, the AI market will expand to $171 billion by 2025,2 or fourfold from $40 billion in 2020.
But while the first quarter of 2022 hit an all-time high for AI investments, private company valuations have dropped following the public market downturn in 2022. For a business to continue to raise capital in these tighter markets, it will have to prove it’s viable.
The most successful companies will be the ones that show how they can apply their AI solutions repeatedly in a productized fashion, providing value above and beyond legacy technology methods. What’s more, organizations with the “Explainable AI” component built into their business models will enhance trust and, in turn, be the ones with the clearest roads to success. People want to see real results, whether in the form of financial performance, customer experience improvements, and market penetration – all metrics that add up to success, and ROI.
A Decade of Adoption: As one of the fastest-growing technology investment banks on Wall Street1, we’ve had a birds-eye view of how the AI landscape has evolved and performed. With the technology adoption curve getting shorter and shorter, we think that most companies will be using far more sophisticated AI technologies within the next decade.
In light of the AI and the database space maturing, our goal is to help the companies we work with take their innovations forward so that they can provide value to their clients. As mentioned, the ROI cases in AI investments are plenty - time and cost savings, productivity and revenue increases, improvement in experience and retention and organizational agility, to name a few. As the technology continues to improve and the cost of adoption decreases, the value proposition of these AI and new database tools will only increase. Therefore, organizations must stay focused on the future and continue betting on AI.
1 Bloomberg
2 GlobeNewswire